DoubleMLパッケージによるDMLのシミュレーション#
参考:Python: Basics of Double Machine Learning — DoubleML documentation
import warnings
import numpy as np
from scipy import stats
import matplotlib.pyplot as plt
import seaborn as sns
from lightgbm import LGBMRegressor
from sklearn.ensemble import RandomForestRegressor
from sklearn.model_selection import train_test_split
from sklearn.base import clone
from doubleml import DoubleMLData
from doubleml import DoubleMLPLR
from doubleml.datasets import make_plr_CCDDHNR2018
face_colors = sns.color_palette('pastel')
edge_colors = sns.color_palette('dark')
warnings.filterwarnings("ignore")
np.random.seed(1234)
n_rep = 1000
n_obs = 500
n_vars = 5
alpha = 0.5
data = list()
for i_rep in range(n_rep):
(x, y, d) = make_plr_CCDDHNR2018(alpha=alpha, n_obs=n_obs, dim_x=n_vars, return_type='array')
data.append((x, y, d))
naiive estimator#
\[
\hat{\theta}_0 = \left(\frac{1}{n} \sum_{i\in I} D_i^2\right)^{-1} \frac{1}{n} \sum_{i\in I} D_i (Y_i - \hat{g}_0(X_i)).
\]
def non_orth_score(y, d, l_hat, m_hat, g_hat, smpls):
u_hat = y - g_hat
psi_a = -np.multiply(d, d)
psi_b = np.multiply(d, u_hat)
return psi_a, psi_b
なお、\(\hat{g}_0(X)\)は直接推定できないため間接的に推定している
np.random.seed(1111)
ml_l = LGBMRegressor(n_estimators=300, learning_rate=0.1)
ml_m = LGBMRegressor(n_estimators=300, learning_rate=0.1)
ml_l = RandomForestRegressor(n_estimators=100, max_features=10, max_depth=5, min_samples_leaf=5)
ml_m = RandomForestRegressor(n_estimators=200, max_features=10, max_depth=5, min_samples_leaf=5)
ml_g = clone(ml_l)
theta_nonorth = np.full(n_rep, np.nan)
se_nonorth = np.full(n_rep, np.nan)
for i_rep in range(n_rep):
print(f'Replication {i_rep+1}/{n_rep}', end='\r')
(x, y, d) = data[i_rep]
# choose a random sample for training and estimation
i_train, i_est = train_test_split(np.arange(n_obs), test_size=0.5, random_state=42)
# fit the ML algorithms on the training sample
ml_l.fit(x[i_train, :], y[i_train])
ml_m.fit(x[i_train, :], d[i_train])
psi_a = -np.multiply(d[i_train] - ml_m.predict(x[i_train, :]), d[i_train] - ml_m.predict(x[i_train, :]))
psi_b = np.multiply(d[i_train] - ml_m.predict(x[i_train, :]), y[i_train] - ml_l.predict(x[i_train, :]))
theta_initial = -np.nanmean(psi_b) / np.nanmean(psi_a)
ml_g.fit(x[i_train, :], y[i_train] - theta_initial * d[i_train])
# create out-of-sample predictions
l_hat = ml_l.predict(x[i_est, :])
m_hat = ml_m.predict(x[i_est, :])
g_hat = ml_g.predict(x[i_est, :])
external_predictions = {
'd': {
'ml_l': l_hat.reshape(-1, 1),
'ml_m': m_hat.reshape(-1, 1),
'ml_g': g_hat.reshape(-1, 1)
}
}
obj_dml_data = DoubleMLData.from_arrays(x[i_est, :], y[i_est], d[i_est])
obj_dml_plr_nonorth = DoubleMLPLR(obj_dml_data,
ml_l, ml_m, ml_g,
n_folds=2,
score=non_orth_score)
obj_dml_plr_nonorth.fit(external_predictions=external_predictions)
theta_nonorth[i_rep] = obj_dml_plr_nonorth.coef[0]
se_nonorth[i_rep] = obj_dml_plr_nonorth.se[0]
fig_non_orth, ax = plt.subplots(constrained_layout=True);
ax = sns.histplot((theta_nonorth - alpha)/se_nonorth,
color=face_colors[0], edgecolor = edge_colors[0],
stat='density', bins=30, label='Non-orthogonal ML');
ax.axvline(0., color='k');
xx = np.arange(-5, +5, 0.001)
yy = stats.norm.pdf(xx)
ax.plot(xx, yy, color='k', label='$\\mathcal{N}(0, 1)$');
ax.legend(loc='upper right', bbox_to_anchor=(1.2, 1.0));
ax.set_xlim([-6., 6.]);
ax.set_xlabel('$(\hat{\\theta}_0 - \\theta_0)/\hat{\sigma}$');
plt.show()
Replication 1/1000
Replication 2/1000
Replication 3/1000
Replication 4/1000
Replication 5/1000
Replication 6/1000
Replication 7/1000
Replication 8/1000
Replication 9/1000
Replication 10/1000
Replication 11/1000
Replication 12/1000
Replication 13/1000
Replication 14/1000
Replication 15/1000
Replication 16/1000
Replication 17/1000
Replication 18/1000
Replication 19/1000
Replication 20/1000
Replication 21/1000
Replication 22/1000
Replication 23/1000
Replication 24/1000
Replication 25/1000
Replication 26/1000
Replication 27/1000
Replication 28/1000
Replication 29/1000
Replication 30/1000
Replication 31/1000
Replication 32/1000
Replication 33/1000
Replication 34/1000
Replication 35/1000
Replication 36/1000
Replication 37/1000
Replication 38/1000
Replication 39/1000
Replication 40/1000
Replication 41/1000
Replication 42/1000
Replication 43/1000
Replication 44/1000
Replication 45/1000
Replication 46/1000
Replication 47/1000
Replication 48/1000
Replication 49/1000
Replication 50/1000
Replication 51/1000
Replication 52/1000
Replication 53/1000
Replication 54/1000
Replication 55/1000
Replication 56/1000
Replication 57/1000
Replication 58/1000
Replication 59/1000
Replication 60/1000
Replication 61/1000
Replication 62/1000
Replication 63/1000
Replication 64/1000
Replication 65/1000
Replication 66/1000
Replication 67/1000
Replication 68/1000
Replication 69/1000
Replication 70/1000
Replication 71/1000
---------------------------------------------------------------------------
KeyboardInterrupt Traceback (most recent call last)
Cell In[3], line 21
18 i_train, i_est = train_test_split(np.arange(n_obs), test_size=0.5, random_state=42)
20 # fit the ML algorithms on the training sample
---> 21 ml_l.fit(x[i_train, :], y[i_train])
22 ml_m.fit(x[i_train, :], d[i_train])
24 psi_a = -np.multiply(d[i_train] - ml_m.predict(x[i_train, :]), d[i_train] - ml_m.predict(x[i_train, :]))
File /usr/local/lib/python3.10/site-packages/sklearn/base.py:1473, in _fit_context.<locals>.decorator.<locals>.wrapper(estimator, *args, **kwargs)
1466 estimator._validate_params()
1468 with config_context(
1469 skip_parameter_validation=(
1470 prefer_skip_nested_validation or global_skip_validation
1471 )
1472 ):
-> 1473 return fit_method(estimator, *args, **kwargs)
File /usr/local/lib/python3.10/site-packages/sklearn/ensemble/_forest.py:478, in BaseForest.fit(self, X, y, sample_weight)
473 if self.warm_start and len(self.estimators_) > 0:
474 # We draw from the random state to get the random state we
475 # would have got if we hadn't used a warm_start.
476 random_state.randint(MAX_INT, size=len(self.estimators_))
--> 478 trees = [
479 self._make_estimator(append=False, random_state=random_state)
480 for i in range(n_more_estimators)
481 ]
483 # Parallel loop: we prefer the threading backend as the Cython code
484 # for fitting the trees is internally releasing the Python GIL
485 # making threading more efficient than multiprocessing in
486 # that case. However, for joblib 0.12+ we respect any
487 # parallel_backend contexts set at a higher level,
488 # since correctness does not rely on using threads.
489 trees = Parallel(
490 n_jobs=self.n_jobs,
491 verbose=self.verbose,
(...)
507 for i, t in enumerate(trees)
508 )
File /usr/local/lib/python3.10/site-packages/sklearn/ensemble/_forest.py:479, in <listcomp>(.0)
473 if self.warm_start and len(self.estimators_) > 0:
474 # We draw from the random state to get the random state we
475 # would have got if we hadn't used a warm_start.
476 random_state.randint(MAX_INT, size=len(self.estimators_))
478 trees = [
--> 479 self._make_estimator(append=False, random_state=random_state)
480 for i in range(n_more_estimators)
481 ]
483 # Parallel loop: we prefer the threading backend as the Cython code
484 # for fitting the trees is internally releasing the Python GIL
485 # making threading more efficient than multiprocessing in
486 # that case. However, for joblib 0.12+ we respect any
487 # parallel_backend contexts set at a higher level,
488 # since correctness does not rely on using threads.
489 trees = Parallel(
490 n_jobs=self.n_jobs,
491 verbose=self.verbose,
(...)
507 for i, t in enumerate(trees)
508 )
File /usr/local/lib/python3.10/site-packages/sklearn/ensemble/_base.py:145, in BaseEnsemble._make_estimator(self, append, random_state)
139 def _make_estimator(self, append=True, random_state=None):
140 """Make and configure a copy of the `estimator_` attribute.
141
142 Warning: This method should be used to properly instantiate new
143 sub-estimators.
144 """
--> 145 estimator = clone(self.estimator_)
146 estimator.set_params(**{p: getattr(self, p) for p in self.estimator_params})
148 if random_state is not None:
File /usr/local/lib/python3.10/site-packages/sklearn/base.py:90, in clone(estimator, safe)
41 """Construct a new unfitted estimator with the same parameters.
42
43 Clone does a deep copy of the model in an estimator
(...)
87 False
88 """
89 if hasattr(estimator, "__sklearn_clone__") and not inspect.isclass(estimator):
---> 90 return estimator.__sklearn_clone__()
91 return _clone_parametrized(estimator, safe=safe)
File /usr/local/lib/python3.10/site-packages/sklearn/base.py:296, in BaseEstimator.__sklearn_clone__(self)
295 def __sklearn_clone__(self):
--> 296 return _clone_parametrized(self)
File /usr/local/lib/python3.10/site-packages/sklearn/base.py:121, in _clone_parametrized(estimator, safe)
113 raise TypeError(
114 "Cannot clone object '%s' (type %s): "
115 "it does not seem to be a scikit-learn "
116 "estimator as it does not implement a "
117 "'get_params' method." % (repr(estimator), type(estimator))
118 )
120 klass = estimator.__class__
--> 121 new_object_params = estimator.get_params(deep=False)
122 for name, param in new_object_params.items():
123 new_object_params[name] = clone(param, safe=False)
File /usr/local/lib/python3.10/site-packages/sklearn/base.py:243, in BaseEstimator.get_params(self, deep)
228 """
229 Get parameters for this estimator.
230
(...)
240 Parameter names mapped to their values.
241 """
242 out = dict()
--> 243 for key in self._get_param_names():
244 value = getattr(self, key)
245 if deep and hasattr(value, "get_params") and not isinstance(value, type):
File /usr/local/lib/python3.10/site-packages/sklearn/base.py:208, in BaseEstimator._get_param_names(cls)
204 return []
206 # introspect the constructor arguments to find the model parameters
207 # to represent
--> 208 init_signature = inspect.signature(init)
209 # Consider the constructor parameters excluding 'self'
210 parameters = [
211 p
212 for p in init_signature.parameters.values()
213 if p.name != "self" and p.kind != p.VAR_KEYWORD
214 ]
File /usr/local/lib/python3.10/inspect.py:3254, in signature(obj, follow_wrapped, globals, locals, eval_str)
3252 def signature(obj, *, follow_wrapped=True, globals=None, locals=None, eval_str=False):
3253 """Get a signature object for the passed callable."""
-> 3254 return Signature.from_callable(obj, follow_wrapped=follow_wrapped,
3255 globals=globals, locals=locals, eval_str=eval_str)
File /usr/local/lib/python3.10/inspect.py:3002, in Signature.from_callable(cls, obj, follow_wrapped, globals, locals, eval_str)
2998 @classmethod
2999 def from_callable(cls, obj, *,
3000 follow_wrapped=True, globals=None, locals=None, eval_str=False):
3001 """Constructs Signature for the given callable object."""
-> 3002 return _signature_from_callable(obj, sigcls=cls,
3003 follow_wrapper_chains=follow_wrapped,
3004 globals=globals, locals=locals, eval_str=eval_str)
File /usr/local/lib/python3.10/inspect.py:2463, in _signature_from_callable(obj, follow_wrapper_chains, skip_bound_arg, globals, locals, eval_str, sigcls)
2458 return sig.replace(parameters=new_params)
2460 if isfunction(obj) or _signature_is_functionlike(obj):
2461 # If it's a pure Python function, or an object that is duck type
2462 # of a Python function (Cython functions, for instance), then:
-> 2463 return _signature_from_function(sigcls, obj,
2464 skip_bound_arg=skip_bound_arg,
2465 globals=globals, locals=locals, eval_str=eval_str)
2467 if _signature_is_builtin(obj):
2468 return _signature_from_builtin(sigcls, obj,
2469 skip_bound_arg=skip_bound_arg)
File /usr/local/lib/python3.10/inspect.py:2370, in _signature_from_function(cls, func, skip_bound_arg, globals, locals, eval_str)
2365 parameters.append(Parameter(name, annotation=annotation,
2366 kind=_VAR_KEYWORD))
2368 # Is 'func' is a pure Python function - don't validate the
2369 # parameters list (for correct order and defaults), it should be OK.
-> 2370 return cls(parameters,
2371 return_annotation=annotations.get('return', _empty),
2372 __validate_parameters__=is_duck_function)
KeyboardInterrupt:
Overcoming regularization bias by orthogonalization#
orthogonalized regressor \(V=D-m(X)\)を用いる推定量
\[
\check{\theta}_0 = \left(\frac{1}{n} \sum_{i\in I} \hat{V}_i D_i\right)^{-1} \frac{1}{n} \sum_{i\in I} \hat{V}_i (Y_i - \hat{g}_0(X_i)).
\]
np.random.seed(2222)
theta_orth_nosplit = np.full(n_rep, np.nan)
se_orth_nosplit = np.full(n_rep, np.nan)
for i_rep in range(n_rep):
print(f'Replication {i_rep+1}/{n_rep}', end='\r')
(x, y, d) = data[i_rep]
# fit the ML algorithms on the training sample
ml_l.fit(x, y)
ml_m.fit(x, d)
psi_a = -np.multiply(d - ml_m.predict(x), d - ml_m.predict(x))
psi_b = np.multiply(d - ml_m.predict(x), y - ml_l.predict(x))
theta_initial = -np.nanmean(psi_b) / np.nanmean(psi_a)
ml_g.fit(x, y - theta_initial * d)
l_hat = ml_l.predict(x)
m_hat = ml_m.predict(x)
g_hat = ml_g.predict(x)
external_predictions = {
'd': {
'ml_l': l_hat.reshape(-1, 1),
'ml_m': m_hat.reshape(-1, 1),
'ml_g': g_hat.reshape(-1, 1)
}
}
obj_dml_data = DoubleMLData.from_arrays(x, y, d)
obj_dml_plr_orth_nosplit = DoubleMLPLR(obj_dml_data,
ml_l, ml_m, ml_g,
score='IV-type')
obj_dml_plr_orth_nosplit.fit(external_predictions=external_predictions)
theta_orth_nosplit[i_rep] = obj_dml_plr_orth_nosplit.coef[0]
se_orth_nosplit[i_rep] = obj_dml_plr_orth_nosplit.se[0]
fig_orth_nosplit, ax = plt.subplots(constrained_layout=True);
ax = sns.histplot((theta_orth_nosplit - alpha)/se_orth_nosplit,
color=face_colors[1], edgecolor = edge_colors[1],
stat='density', bins=30, label='Double ML (no sample splitting)');
ax.axvline(0., color='k');
xx = np.arange(-5, +5, 0.001)
yy = stats.norm.pdf(xx)
ax.plot(xx, yy, color='k', label='$\\mathcal{N}(0, 1)$');
ax.legend(loc='upper right', bbox_to_anchor=(1.2, 1.0));
ax.set_xlim([-6., 6.]);
ax.set_xlabel('$(\hat{\\theta}_0 - \\theta_0)/\hat{\sigma}$');
plt.show()
Replication 1000/1000
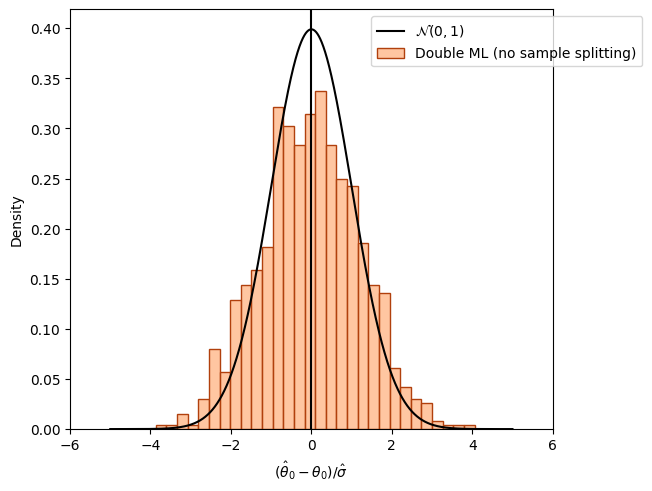
Sample splitting to remove bias induced by overfitting#
np.random.seed(3333)
theta_dml = np.full(n_rep, np.nan)
se_dml = np.full(n_rep, np.nan)
for i_rep in range(n_rep):
print(f'Replication {i_rep+1}/{n_rep}', end='\r')
(x, y, d) = data[i_rep]
obj_dml_data = DoubleMLData.from_arrays(x, y, d)
obj_dml_plr = DoubleMLPLR(obj_dml_data,
ml_l, ml_m, ml_g,
n_folds=2,
score='IV-type')
obj_dml_plr.fit()
theta_dml[i_rep] = obj_dml_plr.coef[0]
se_dml[i_rep] = obj_dml_plr.se[0]
fig_dml, ax = plt.subplots(constrained_layout=True);
ax = sns.histplot((theta_dml - alpha)/se_dml,
color=face_colors[2], edgecolor = edge_colors[2],
stat='density', bins=30, label='Double ML with cross-fitting');
ax.axvline(0., color='k');
xx = np.arange(-5, +5, 0.001)
yy = stats.norm.pdf(xx)
ax.plot(xx, yy, color='k', label='$\\mathcal{N}(0, 1)$');
ax.legend(loc='upper right', bbox_to_anchor=(1.2, 1.0));
ax.set_xlim([-6., 6.]);
ax.set_xlabel('$(\hat{\\theta}_0 - \\theta_0)/\hat{\sigma}$');
plt.show()
Replication 1000/1000
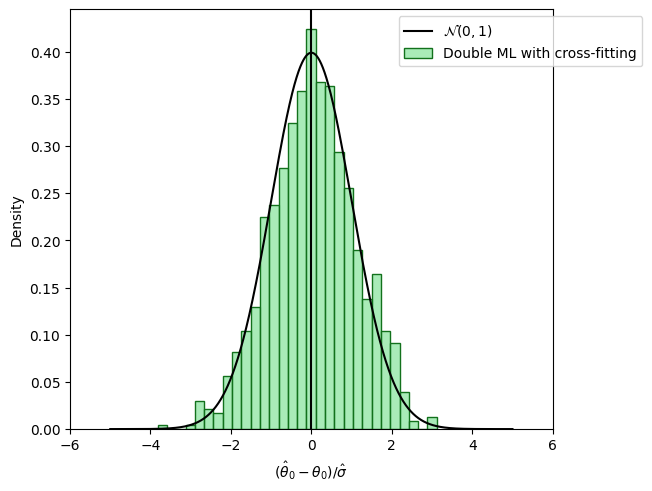