Double/Debiased Machine Learning#
Python Package: DoubleML
概要#
世の中の多くの現象は非線形な関係性が想定される。回帰分析は線形モデルであるため、モデルの定式化の誤りに起因するバイアスが生じかねない。
実際に関心のあるパラメータは少なく、交絡のコントロールのために入れている局外母数(nuisance parameters)は高次元になりがち。
局外母数を非線形の関数
を作り、局外関数
もしこれが実現できれば、非線形部分を機械学習による優れた関数近似性能で捉えて交絡をコントロールしつつ、パラメータは線形回帰モデルのように推定できる。
これを実現するのがDMLのフレームワークである(
DML の大まかなイメージ#
DML の論文の提案手法・主要な貢献は
ネイマン直交性(Neyman orthogonality) という性質を持つスコア関数によるモーメント法が、機械学習を用いたモデルに
一致推定量をもたらすことCross-Validation のようにサンプルを分割した Cross-Fitting による推定がよいこと
を示したこと。
ネイマン直交性をもつスコア関数による推定量の例は、
によって
その際に過学習していると残差が過小評価されるので、「訓練用データと残差の評価用のデータをランダムに分割して推定することを
DML 自体は抽象化されたフレームワークだが、具体例としては上記のように「残差回帰を分割したサンプルで
先行研究#
Robinson (1988) は部分線形モデルを提案し、一致推定量も導出した
Robinson (1988)の推定量
部分線形モデル
の両辺を
これをモデルから差し引くと
という線形回帰の形になる(FWL定理の残差回帰の形)
ただし、
その推定量はroot-N consistentで漸近正規性を持つ
参考
西山・人見(2023)『ノン・セミパラメトリック統計解析』、共立出版
Robinson, P. M. (1988). Root‐N‐consistent semi‐parametric regression. Econometrica 56, 931–54.
Donsker条件#
Andrew (1994) はプラグイン推定量が漸近正規性・
その条件を満たすために使われるのが、Donsker条件(Donsker condition)という条件を満たすクラスの関数(複雑性の低い関数)をノンパラメトリック推定量に使うというもの。
機械学習アルゴリズムによる推定量は複雑度が高く、一般にDonsker条件を満たさない。よってRobinson(1988)の推定量に機械学習をそのまま組み込むと漸近正規推定量・
課題#
2つのバイアスがある
正則化バイアス(Reguralization bias)
過学習によるバイアス(Bias induced by overfifting)
それぞれの対策として、
正則化バイアス → ネイマン直交化(例:残差回帰) で対応
過学習 → Cross-Fitting で対応
を行う
前提知識#
知ってると理解を深めやすくなる前提知識まとめ
(参考)残差回帰
DMLでは、FWL定理を利用した残差回帰を一般化する。
残差回帰は線形回帰モデル
を用いて
のようにして関心のあるパラメータ
線形回帰モデルは回帰関数
と表すことができる。
FWL定理
目的変数のベクトル
があるとする。
説明変数を
と表す。
この回帰モデルの
を に回帰して残差 を得る: を に回帰して残差 を得る: を に回帰させる:
(参考)モーメント法
確率変数
という条件(直交条件)を満たすスコア関数
を解いて
例:線形回帰モデル
線形回帰モデル
のパラメータ
このモデルから
標本対応は
行列表記では
となる。これを解くと
と、最小二乗法の解と一致する
(参考)漸近理論
確率変数列
分布収束 (converge in distribution)や 法則収束(convergence in law) や 弱収束 (weak convergence) と呼び、
などと表す。
などと表す。
ナイーブな推定量と正則化バイアス#
サンプル分割#
のちのCross Fittingでサンプル分割の話になるので、数式の記法もあらかじめ分割に対応させておく。
main sampleは
補助パートのサンプルで
ナイーブな推定量#
線形回帰モデル
であった。これと同様に部分線形モデルにおいて
というモーメント条件を考えると、その推定量は
となる。この推定量
となる。
この「劣った」振る舞いの背後には
ヒューリスティックにこの
である。
第1項
ヒューリスティックには、
1. The basics of double/debiased machine learning — DoubleML documentation
Show code cell source
import warnings
warnings.filterwarnings("ignore", category=UserWarning)
import numpy as np
from doubleml.datasets import make_plr_CCDDHNR2018
np.random.seed(1234)
n_rep = 1000
n_obs = 500
n_vars = 20
alpha = 0.5
data = list()
for i_rep in range(n_rep):
(x, y, d) = make_plr_CCDDHNR2018(alpha=alpha, n_obs=n_obs, dim_x=n_vars, return_type='array')
data.append((x, y, d))
def non_orth_score(y, d, l_hat, m_hat, g_hat, smpls):
u_hat = y - g_hat
psi_a = -np.multiply(d, d)
psi_b = np.multiply(d, u_hat)
return psi_a, psi_b
from doubleml import DoubleMLData
from doubleml import DoubleMLPLR
from sklearn.ensemble import RandomForestRegressor
from sklearn.base import clone
import numpy as np
from scipy import stats
import matplotlib.pyplot as plt
import seaborn as sns
face_colors = sns.color_palette('pastel')
edge_colors = sns.color_palette('dark')
np.random.seed(1111)
ml_l = RandomForestRegressor(n_estimators=132, max_features=12, max_depth=5, min_samples_leaf=1)
ml_m = RandomForestRegressor(n_estimators=378, max_features=20, max_depth=3, min_samples_leaf=6)
ml_g = clone(ml_l)
# シミュレーションに時間がかかるので結果を保存する
import json
from pathlib import Path
result_json_path = Path('./dml_simulation_results.json')
use_cache = True
# 実験1: ネイマン直交条件を満たさない場合
if use_cache and result_json_path.exists():
with open(result_json_path, "r") as f:
simulation_results = json.load(f)
simulation_results = {k: np.array(v) for k,v in simulation_results.items()}
theta_nonorth = simulation_results["theta_nonorth"]
se_nonorth = simulation_results["se_nonorth"]
else:
theta_nonorth = np.zeros(n_rep)
se_nonorth = np.zeros(n_rep)
for i_rep in range(n_rep):
(x, y, d) = data[i_rep]
obj_dml_data = DoubleMLData.from_arrays(x, y, d)
obj_dml_plr_nonorth = DoubleMLPLR(obj_dml_data,
ml_l, ml_m, ml_g,
n_folds=2,
apply_cross_fitting=False,
score=non_orth_score)
obj_dml_plr_nonorth.fit()
this_theta = obj_dml_plr_nonorth.coef[0]
this_se = obj_dml_plr_nonorth.se[0]
theta_nonorth[i_rep] = this_theta
se_nonorth[i_rep] = this_se
simulation_results = {}
simulation_results["theta_nonorth"] = theta_nonorth
simulation_results["se_nonorth"] = se_nonorth
# plot
plt.figure(constrained_layout=True)
ax = sns.histplot((theta_nonorth - alpha)/se_nonorth,
color=face_colors[0], edgecolor=edge_colors[0],
stat='density', bins=30, label='Non-orthogonal ML')
ax.axvline(0., color='k')
xx = np.arange(-5, +5, 0.001)
yy = stats.norm.pdf(xx)
ax.plot(xx, yy, color='k', label='$\\mathcal{N}(0, 1)$')
ax.legend(loc='upper right', bbox_to_anchor=(1.2, 1.0))
ax.set_xlim([-6., 6.])
ax.set_xlabel('$(\hat{\\theta}_0 - \\theta_0)/\hat{\sigma}$')
plt.show()
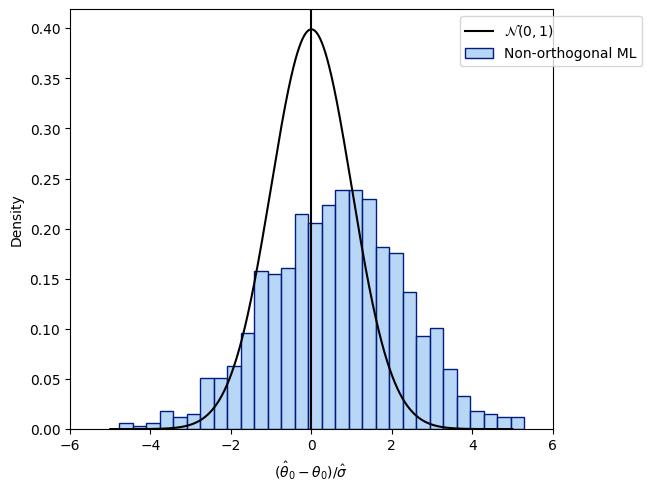
直交化による正則化バイアスの打破#
別の推定量として、モーメント条件
を用いる場合を考える。先程との違いは、DからXの効果をpartialling outして直交化された
を使用する点である。ここで
DからXの効果をpartialling out したあとは、main sampleを使って
この推定量
近似的にDをXについて直交化し、
DML:
IV:
Show code cell source
# 実験2: 直交化した場合
if use_cache and result_json_path.exists():
with open(result_json_path, "r") as f:
simulation_results = json.load(f)
simulation_results = {k: np.array(v) for k,v in simulation_results.items()}
theta_orth_nosplit = simulation_results["theta_orth_nosplit"]
se_orth_nosplit = simulation_results["se_orth_nosplit"]
else:
theta_orth_nosplit = np.zeros(n_rep)
se_orth_nosplit = np.zeros(n_rep)
for i_rep in range(n_rep):
(x, y, d) = data[i_rep]
obj_dml_data = DoubleMLData.from_arrays(x, y, d)
obj_dml_plr_orth_nosplit = DoubleMLPLR(obj_dml_data,
ml_l, ml_m, ml_g,
n_folds=1,
score='partialling out',
apply_cross_fitting=False)
obj_dml_plr_orth_nosplit.fit()
this_theta = obj_dml_plr_orth_nosplit.coef[0]
this_se = obj_dml_plr_orth_nosplit.se[0]
theta_orth_nosplit[i_rep] = this_theta
se_orth_nosplit[i_rep] = this_se
simulation_results["theta_orth_nosplit"] = theta_orth_nosplit
simulation_results["se_orth_nosplit"] = se_orth_nosplit
# plot
plt.figure(constrained_layout=True)
ax = sns.histplot((theta_orth_nosplit - alpha)/se_orth_nosplit,
color=face_colors[1], edgecolor=edge_colors[1],
stat='density', bins=30, label='Double ML (no sample splitting)')
ax.axvline(0., color='k')
xx = np.arange(-5, +5, 0.001)
yy = stats.norm.pdf(xx)
ax.plot(xx, yy, color='k', label='$\\mathcal{N}(0, 1)$')
ax.legend(loc='upper right', bbox_to_anchor=(1.2, 1.0))
ax.set_xlim([-6., 6.])
ax.set_xlabel('$(\hat{\\theta}_0 - \\theta_0)/\hat{\sigma}$')
Text(0.5, 0, '$(\\hat{\\theta}_0 - \\theta_0)/\\hat{\\sigma}$')
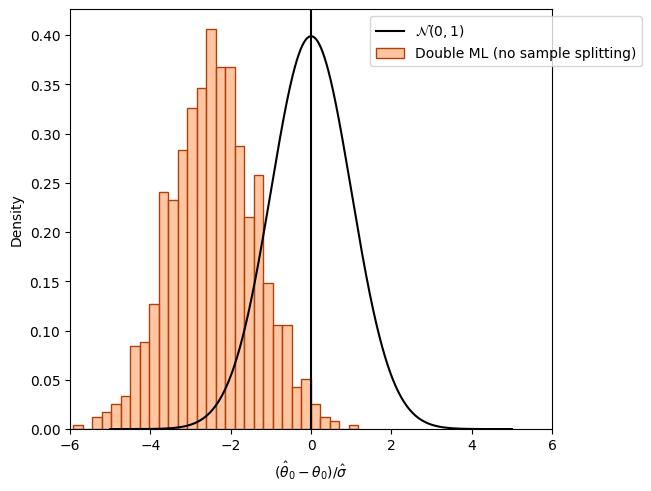
Neyman Orthogonality#
partialling outしたモーメント条件による推定量とナイーブな推定量は何が違うのか?
→ ネイマン直交性(Neyman orthogonality)がカギになる。
ネイマン直交性
サンプル
について、ガトー微分が存在し、微小な
このことを ネイマン直交性(Neyman orthogonality) という
意訳:真の局外母数η0=(m0, g0)と任意のηとの微小な差による直交条件の変化が0であること
→ ηの推定誤差に対して頑健(robust)であること
→ ネイマン直交性をもつスコア関数を用いる推定量は正則化バイアスに対し頑健になる
の性質#
スケールされた推定誤差は3つの要素に分解できる
で、これは
実際、この項は
(多くのMLアルゴリズムが
となる。これが弱い条件のもとで成り立つことを保証するにあたって、sample splittingが重要な役割を果たす。
Cross Fittingによる過学習のバイアスの除去#
DML推定量の
のうち、
などの項を含む。
この項は局外関数の推定誤差と部分線形モデルの構造的未観測要因の積の和を
sample splittingを使うと、このような項をシンプルでタイトにコントロールできる。それを確認するため、観測値が独立と仮定して、
であることがわかり、チェビシェフの不等式を使って確率的には消失することがわかる。
なぜ平均ゼロになるのか
条件付き期待値の性質
ということかと思われる
sample splittingの欠点は推定に使用するサンプル数が減ることによる効率性の低下である。しかし、mainとauxiliaryの2つでそれぞれ推定を行い、両者の平均を取ればfull efficiencyを取り戻す。この手続き(mainとauxiliaryの役割を取り替えて複数の推定値を取得しそれらの平均をとる)を「cross-fitting」と呼ぶことにする。一般にk-foldにすることもできる。
Show code cell source
# 実験2: 直交化した場合
if use_cache and result_json_path.exists():
with open(result_json_path, "r") as f:
simulation_results = json.load(f)
simulation_results = {k: np.array(v) for k,v in simulation_results.items()}
theta_dml = simulation_results["theta_dml"]
se_dml = simulation_results["se_dml"]
else:
theta_dml = np.zeros(n_rep)
se_dml = np.zeros(n_rep)
for i_rep in range(n_rep):
(x, y, d) = data[i_rep]
obj_dml_data = DoubleMLData.from_arrays(x, y, d)
obj_dml_plr = DoubleMLPLR(obj_dml_data,
ml_l, ml_m, ml_g,
n_folds=2,
score='partialling out')
obj_dml_plr.fit()
this_theta = obj_dml_plr.coef[0]
this_se = obj_dml_plr.se[0]
theta_dml[i_rep] = this_theta
se_dml[i_rep] = this_se
simulation_results["theta_dml"] = theta_dml
simulation_results["se_dml"] = se_dml
simulation_results = {k:list(v) for k,v in simulation_results.items()}
with open(result_json_path, "w") as f:
json.dump(simulation_results, f)
# plot
plt.figure(constrained_layout=True)
ax = sns.histplot((theta_dml - alpha)/se_dml,
color=face_colors[2], edgecolor = edge_colors[2],
stat='density', bins=30, label='Double ML with cross-fitting')
ax.axvline(0., color='k')
xx = np.arange(-5, +5, 0.001)
yy = stats.norm.pdf(xx)
ax.plot(xx, yy, color='k', label='$\\mathcal{N}(0, 1)$')
ax.legend(loc='upper right', bbox_to_anchor=(1.2, 1.0))
ax.set_xlim([-6., 6.])
ax.set_xlabel('$(\hat{\\theta}_0 - \\theta_0)/\hat{\sigma}$')
plt.show()
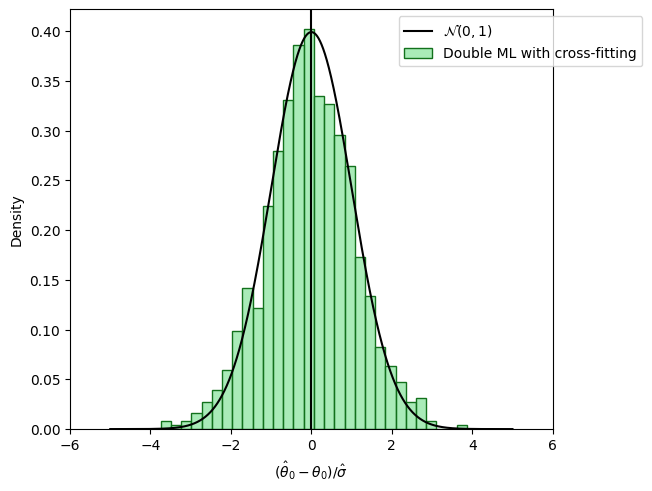
Cross Fitting#
Definition 3.1.DML1
(1) サンプル
それぞれの
(2) 各
を構築する。
(3) 各
の解として構築する。
である。
なお、もし厳密に0にするのが不可能である場合は、推定量
ここで
(4) 推定量を集計する
DML1(簡略版)
サンプル
のインデックス のK-foldのランダムな分割 を作る。 のサイズは である。それぞれの
について、 を定義する。各
について、局外母数 のML推定量を構築する。
各
について、推定量 をの解として構築する。
はネイマン直交スコアである。推定量を集計する
DML2(簡略版)
サンプル
のインデックス のK-foldのランダムな分割 を作る。 のサイズは である。それぞれの
について、 を定義する。各
について、局外母数 のML推定量を構築する。
各
について、推定量 をの解として構築する。
はネイマン直交スコアである。
We show that if the population risk satisfies a condition called Neyman orthogonality, the impact of the nuisance estimation error on the excess risk bound achieved by the meta-algorithm is of second order.
Donsker条件#
Robinson (1988)などのセミパラメトリックモデルでも
しかし、Donsker条件(Donsker condition)という条件を満たすクラスの関数(複雑性の低い関数)でなければ、漸近正規性をもたない(Andrew 1994)
機械学習モデルの収束レートが遅い(Donsker条件)→Cross Fitting
プラグイン推定量#
モデルのパラメータをモーメント推定する際に、未知の関数をノンパラ推定量で置換してモーメント推定する推定量。 部分線形モデルを含む多くのセミパラメトリック推定量はプラグイン推定量に含まれる。
関心のあるパラメータを
を満たすとする(
を満たすように
プラグイン推定量の漸近正規性#
プラグイン推定量が漸近正規性をもつためには、次の2つの条件が鍵となる
なお
である。
ノンパラメトリック推定量の漸近理論ではDonsker条件を用いて条件1を示すことが多いようだが、高次元ではDonsker条件は満たされない
対処法のひとつは標本分割(sample splitting)だが、標本分割すると効率性が低下する。Chernozkov et al. (2018)ではCross-fittingにより効率性を落とさずに推定できることを示した
の推定#
Robinson-styleのほうは
DMLの
→ 直接推定できないのでちょっと手順を踏む
ドキュメントの例だと
psi_a = -np.multiply(d[i_train] - ml_m.predict(x[i_train, :]), d[i_train] - ml_m.predict(x[i_train, :]))
psi_b = np.multiply(d[i_train] - ml_m.predict(x[i_train, :]), y[i_train] - ml_l.predict(x[i_train, :]))
theta_initial = -np.nanmean(psi_b) / np.nanmean(psi_a)
ml_g.fit(x[i_train, :], y[i_train] - theta_initial * d[i_train])
Remark that the estimator is not able to estimate
directly, but has to be based on a preliminary estimate of Python: Basics of Double Machine Learning — DoubleML documentation
Robinsonのスコア
Robinson (1988)のスコア関数もNeiman orthogonalityを満たし、推定量は残差回帰のような形になるので理解しやすい
を機械学習アルゴリズムで構築し、
という残差で切片なしの単回帰
を行うというもの。
# 実装のイメージ(簡単のためサンプル分割なし)
from lightgbm import LGBMRegressor
m = LGBMRegressor(max_depth=4, verbose=-1)
m.fit(X, D)
l = LGBMRegressor(max_depth=4, verbose=-1)
l.fit(X, Y)
V_hat = D - m.predict(X)
Y_res = Y - l.predict(X)
theta_hat = np.mean(V_hat * V_hat) ** (-1) * np.mean(V_hat * Y_res)
# 実装のイメージ(Cross-fitting)
from sklearn.model_selection import KFold
kf = KFold(n_splits=5)
kf.get_n_splits(X)
thetas = []
for i, (train_idx, test_idx) in enumerate(kf.split(X)):
# 局外関数の推定
m = LGBMRegressor(max_depth=4, verbose=-1).fit(X[train_idx], D[train_idx])
l = LGBMRegressor(max_depth=4, verbose=-1).fit(X[train_idx], Y[train_idx])
# 残差の計算
V_hat = D[test_idx] - m.predict(X[test_idx])
Y_res = Y[test_idx] - l.predict(X[test_idx])
# θの推定
theta_hat = np.mean(V_hat * V_hat) ** (-1) * np.mean(V_hat * Y_res)
thetas.append(theta_hat)
np.mean(thetas)
# 実装のイメージ(簡単のためサンプル分割なし)
from lightgbm import LGBMRegressor
from sklearn.model_selection import KFold
kf = KFold(n_splits=5)
kf.get_n_splits(X)
thetas = []
for i, (train_idx, test_idx) in enumerate(kf.split(X)):
# 局外関数の推定
m = LGBMRegressor(max_depth=4, verbose=-1).fit(X[train_idx], D[train_idx])
l = LGBMRegressor(max_depth=4, verbose=-1).fit(X[train_idx], Y[train_idx])
# 残差の計算
V_hat = D[test_idx] - m.predict(X[test_idx])
Y_res = Y[test_idx] - l.predict(X[test_idx])
# θの推定
theta_hat = np.mean(V_hat * V_hat) ** (-1) * np.mean(V_hat * Y_res)
thetas.append(theta_hat)
np.mean(thetas)
---------------------------------------------------------------------------
NameError Traceback (most recent call last)
Cell In[4], line 6
3 from sklearn.model_selection import KFold
5 kf = KFold(n_splits=5)
----> 6 kf.get_n_splits(X)
7 thetas = []
8 for i, (train_idx, test_idx) in enumerate(kf.split(X)):
9 # 局外関数の推定
NameError: name 'X' is not defined
参考#
参考#
金本拓. (2024). 因果推論: 基礎から機械学習・時系列解析・因果探索を用いた意思決定のアプローチ. 株式会社 オーム社.
22 - Debiased/Orthogonal Machine Learning — Causal Inference for the Brave and True
ノンパラ関連
モーメント法
DMLによるDID#
講義動画(Youtube)#
Double Machine Learning for Causal and Treatment Effects - YouTube
MLでのcausal parametersの推定は良いとは限らない
double or orthogonalized MLとsample splittingによって、causal parametersの高精度な推定が可能
Partially Linear Modelを使う
MLをそのまま使うと一致推定量にならない(例えば
で をRandom Forestで学習しても、予測精度は良いがバイアスがある)FWL定理を用いて、残差の回帰にするとよい
モーメント条件
Regression adjustment:
“propensity score adjustment”:
Neyman-orthogonal (semi-parametrically efficient under homoscedasticity):
3は不偏
Sample Splitting
Splittingによるefficiencyの低下問題
2個に分けて2回やって平均とればfull efficiency → k個に分けての分析をk回やって平均とってもfull efficiency
応用研究#
[2002.08536] Debiased Off-Policy Evaluation for Recommendation Systems
関連研究#
先行研究まとめがある
[2008.06461] Estimating Structural Target Functions using Machine Learning and Influence Functions
Influence Function Learningという新しいフレームワークを提案
Library Flow Chart — econml 0.15.0 documentation
派生モデルの使い分けについて
部分線形モデルに対するモーメント条件#
異なる推定量でどれだけバイアスが入るか確認したい
import numpy as np
def g(X):
# linear
return 2 * X
n = 1000
np.random.seed(0)
X = np.random.uniform(size=n)
theta = 3
D = X + np.random.normal(size=n)
Y = D * theta + g(X) + np.random.normal(size=n)
import pandas as pd
df = pd.DataFrame({"Y": Y, "D": D, "X": X})
X_mat = X.reshape(-1, 1)
D_mat = D.reshape(-1, 1)
ナイーブな推定量
かりに、
from lightgbm import LGBMRegressor
# Y ~ X
g = LGBMRegressor(max_depth=4, verbose=-1)
g.fit(X_mat, Y)
# Y_res := Y - g(X)
Y_res = Y - g.predict(X_mat)
theta_hat = np.mean(D**2) ** (-1) * np.mean(D * Y_res)
print(f"θ={theta_hat:.3f}")
# Y_res ~ D のOLS
import statsmodels.formula.api as smf
final_model = smf.ols(
formula='Y_res ~ -1 + D',
data=df.assign(
Y_res = Y - g.predict(X_mat)
)
).fit()
final_model.summary().tables[1]
θ=1.981
coef | std err | t | P>|t| | [0.025 | 0.975] | |
---|---|---|---|---|---|---|
D | 1.9811 | 0.049 | 40.446 | 0.000 | 1.885 | 2.077 |
from sklearn.linear_model import LinearRegression
# gをDonsker条件を満たしそうな線形モデルにしたらどうなるか
# これは普通に残渣回帰でもないしだめか
# Y ~ X
g = LinearRegression()
g.fit(X_mat, Y)
# Y_res := Y - g(X)
Y_res = Y - g.predict(X_mat)
theta_hat = np.mean(D**2) ** (-1) * np.mean(D * Y_res)
print(f"θ={theta_hat:.3f}")
θ=2.177
g.coef_
array([4.65807719])
# TODO: モーメント条件の方向微分をplotできないものか?
# 横軸はthetaとr
DML score function#
ネイマン直交性を満たす
from lightgbm import LGBMRegressor
from sklearn.model_selection import cross_val_predict
# Y ~ X
g = LGBMRegressor(max_depth=4, verbose=-1)
g.fit(X_mat, Y)
# D ~ X
m = LGBMRegressor(max_depth=4, verbose=-1)
m.fit(X_mat, D)
# y_res := Y - g(X)
# V_hat := D - m(X)
V_hat = D - m.predict(X_mat)
Y_res = Y - g.predict(X_mat)
theta_hat = np.mean(V_hat * D) ** (-1) * np.mean(V_hat * Y_res)
print(f"θ={theta_hat:.3f}")
import statsmodels.formula.api as smf
final_model = smf.ols(
formula='Y_res ~ -1 + V_hat',
data=df.assign(
Y_res = Y_res,
V_hat = V_hat,
)
).fit()
final_model.summary().tables[1]
θ=2.872
coef | std err | t | P>|t| | [0.025 | 0.975] | |
---|---|---|---|---|---|---|
V_hat | 2.9672 | 0.032 | 93.561 | 0.000 | 2.905 | 3.029 |
Robinson-style “partialling-out” score function#
部分線形モデル
の両辺を
これをモデルから差し引くと
という線形回帰の形になる。
ただし、
こちらもネイマン直交性を満たす。
DMLの論文のSection 4.1でRobinsonのスコア関数もネイマン直交性を満たすことを述べているが、DMLのスコア関数が優れてるなどといった説明はとくに無いようだった。
cross fitting#
# cross-fittingあり
from lightgbm import LGBMRegressor
from sklearn.model_selection import cross_val_predict
m = LGBMRegressor(max_depth=6, verbose=-1)
df["V_hat"] = df["D"] - cross_val_predict(m, df[["X"]], df["D"], cv=5)
g = LGBMRegressor(max_depth=6, verbose=-1)
df["Y_res"] = df["Y"] - cross_val_predict(g, df[["X"]], df["Y"], cv=5)
import statsmodels.formula.api as smf
final_model = smf.ols(formula='Y_res ~ -1 + V_hat', data=df).fit()
final_model.summary().tables[1]
coef | std err | t | P>|t| | [0.025 | 0.975] | |
---|---|---|---|---|---|---|
V_hat | 2.9471 | 0.032 | 92.429 | 0.000 | 2.885 | 3.010 |