分位点回帰#
分位点\(\tau\)における条件付分位関数を
と表す。ここで\(F_y^{-1}(\tau | X_i)\)は\(y\)において\(X_i\)に条件づけられた\(y_i\)の分布関数である(\(F_y^{-1}(\tau | X_i) = \inf \{ y: F_y(y|X_i) \geq \tau \}\))。
例えば\(\tau = 0.1\)のとき、\(Q_\tau(y_i | X_i)\)は\(y_i\)の下位10分位である。
標準的な回帰モデルは二乗誤差\((y_i - m(X_i))^2\)の和や期待値を最小化するようにモデル\(m(X_i)\)を学習して条件付き期待値\(E(y_i|X_i)\)を予測する
分位点回帰 (quantile regression)モデルはpinball loss\(\rho_{\tau}(y_i - q(X_i))\)の和や期待値を最小化するようにモデル\(q(X_i)\)を学習させ、条件付き分位関数\(Q_{\tau}(y_i|X_i) = F^{-1}_y(\tau|X_i)\)を予測する
pinball lossは \(\tau\)-tiled absolute value function や 検定関数(check function)とも呼ばれる(グラフを描くとチェックマークに似てるため)
あるいは
あるいは
と書かれる
Show code cell source
import numpy as np
import matplotlib.pyplot as plt
def pinball_loss(x, tau):
return (tau - 1 * (x <= 0)) * x
x = np.linspace(-1, 1, 100)
fig, axes = plt.subplots(figsize=[10, 2], ncols=3)
for i, tau in enumerate([0.1, 0.5, 0.9]):
y = pinball_loss(x, tau=tau)
axes[i].plot(x, y)
if i == 0:
axes[i].set(title=f"τ={tau}", xlabel=r"$x$", ylabel=r"$y = (\tau - 1(x <= 0)) x$")
else:
axes[i].set(title=f"τ={tau}", xlabel=r"$x$")
fig.show()
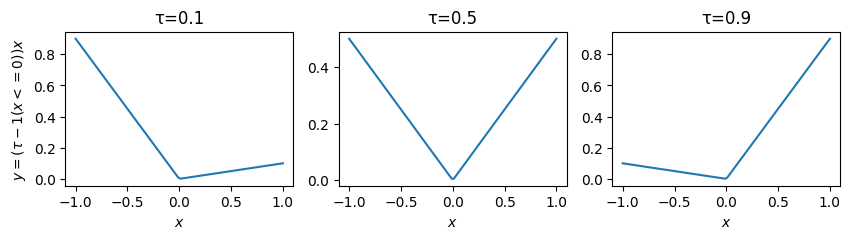
なお、pinball lossは\(\tau=0.5\)のとき
と、絶対誤差と比例する形になる。
絶対誤差の和を目的関数にとった線形モデルは統計学においてleast absolute deviations (LAD) と呼ばれ、その解は条件付き中央値になる
Show code cell source
import numpy as np
import matplotlib.pyplot as plt
def pinball_loss(x, tau):
return (tau - 1 * (x <= 0)) * x
x = np.linspace(-3, 3, 100)
fig, ax = plt.subplots(figsize=[4, 3])
ax.plot(x, pinball_loss(x, tau=0.5), label=r"$\rho_{0.5}(x)$")
ax.plot(x, abs(x), label="|x|")
ax.legend()
fig.show()
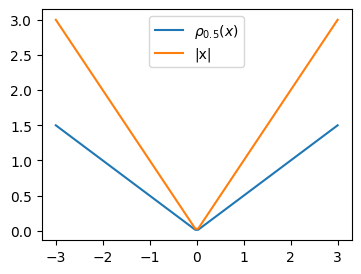
メモ:誤差と最適解#
二乗誤差の場合#
二乗誤差
の場合、
と変形すると、\(E(y|x) = f(x)\)のときに最小化され、予測値\(f(x)\)を含まない第1項のみが残ることがわかる
絶対誤差の場合#
絶対誤差
3.3. Metrics and scoring: quantifying the quality of predictions — scikit-learn 1.4.1 documentation
分位点回帰モデルの実践#
LightGBMでのquantile regression#
目的関数をbinball lossにすればいいだけなので他のアルゴリズムでも実行できる
Show code cell source
import numpy as np
import matplotlib.pyplot as plt
# create data
np.random.seed(0)
n = 1000
x = np.random.uniform(-3, 3, size=n)
X = x.reshape(-1, 1)
y = np.sin(x) + np.random.normal(scale=0.5, size=n)
fig, ax = plt.subplots()
ax.scatter(x, y, alpha=0.7)
# regression
x_plot = np.linspace(-3, 3, 500)
X_test = x_plot.reshape(-1, 1)
import lightgbm as lgb
taus = [0.1, 0.9]
colors = ["orange", "tomato"]
for i in range(2):
model = lgb.LGBMRegressor(objective="quantile", alpha=taus[i], verbose=-1)
model.fit(X, y)
y_hat = model.predict(X_test)
ax.plot(X_test, y_hat, color=colors[i], label=fr"$\tau = {taus[i]}$")
ax.set(xlabel="x", ylabel="y", title="Quantile Regression with LightGBM")
ax.legend()
fig.show()
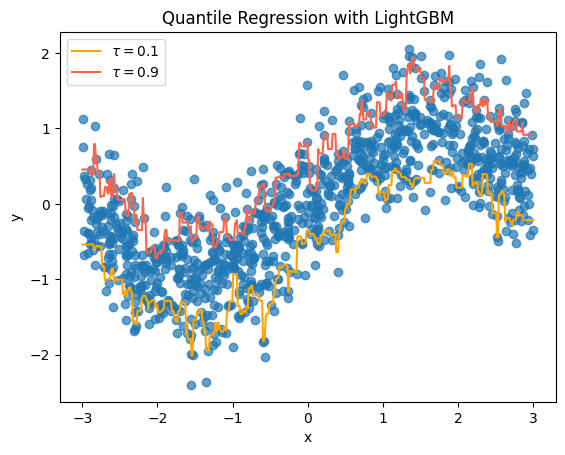
from sklearn.metrics import d2_pinball_score, make_scorer
d2_pinball_score_09 = make_scorer(d2_pinball_score, alpha=0.9)
d2_pinball_score_09(model, X, y)
0.48475107952573926