概要#
単回帰モデル#
モデル#
説明変数
これに対する次のようなモデルを単回帰モデルという。
パラメータの推定#
通常最小二乗法(ordinary least squares: OLS)という方法を使うのが最も一般的である。これは実測値
を最小化するようにパラメータを推定するというもの。
この最適化は、まずRSSをパラメータ
これを整理すると
となる。
式展開メモ
ひとつめの式
を整理すると
整理すると
2つ目の式
は整理すると
1行目の両辺に
を2行目から差し引くと
両辺に
少し整理すると
を
Note
最尤推定量(単回帰モデル)
残差二乗和(RSS)の等高線を描いてみると、OLS推定値が極小値となっていることがわかる。(OLS以外のExamplesは適当な値を入れた単なる例。最適解からズレていることがわかる。)
Show code cell source
import numpy as np
import pandas as pd
import matplotlib.pyplot as plt
import seaborn as sns
# data -------------------------
np.random.seed(0)
n = 10
beta_0 = 5
beta_1 = 10
x = np.sort(np.random.uniform(size=n))
e = np.random.normal(scale=2, size=n)
y = beta_0 + beta_1 * x + e
# calc RSS ---------------------
def rss(y_true, x, beta_0, beta_1):
y_pred = beta_0 + beta_1 * x
return sum((y_true - y_pred)**2)
beta_0_range = np.linspace(-5, 20, 26)
beta_1_range = np.linspace(-5, 20, 26)
Beta0, Beta1 = np.meshgrid(beta_0_range, beta_1_range)
Loss = np.array([rss(y, x, b0, b1) for b0, b1 in zip(Beta0.flatten(), Beta1.flatten())]).reshape(Beta0.shape)
# OLS estimate ------------------
beta_1_hat = np.cov(x, y)[0, 1] / np.var(x)
beta_0_hat = y.mean() - beta_1_hat * x.mean()
loss_ = rss(y, x, beta_0_hat, beta_1_hat)
color_ols = "black"
# wrong estimate
beta_wrongs = [(15, -4), (5, 9)]
color_wrong = "red"
# plot ---------------------------
fig, axes = plt.subplots(ncols=2, figsize=[10,3])
# scatter
axes[0].scatter(x, y)
axes[0].set(xlabel="x", ylabel="y", title="example of linear models")
for i, (b0, b1) in enumerate(beta_wrongs, 1):
y_pred = b0 + b1 * x
axes[0].plot(x, y_pred, label=f"Example {i}: β0={b0:.1f}, β1={b1:.1f}", color=color_wrong, linestyle=":", alpha=.5)
y_pred = beta_0_hat + beta_1_hat * x
axes[0].plot(x, y_pred, label=f"OLS Estimates: β0={beta_0_hat:.1f}, β1={beta_1_hat:.1f}", color=color_ols)
axes[0].legend(loc="lower right")
# RSS
cs = axes[1].contourf(Beta0, Beta1, Loss, levels=[0, 50, 100, 200], alpha=0.5)
axes[1].set(xlabel="β0", ylabel="β1", title="Residual Sum of Squares")
for i, (b0, b1) in enumerate(beta_wrongs, 1):
axes[1].scatter(b0, b1, color=color_wrong)
axes[1].text(b0 * 1.1, b1, f"Example {i}\n(β0={b0:.1f}, β1={b1:.1f})", color=color_wrong, alpha=.5)
axes[1].scatter(beta_0_hat, beta_1_hat, color=color_ols)
axes[1].text(beta_0_hat * 1.1, beta_1_hat, f"Minimum Point (OLS Estimates)\n(β0={beta_0_hat:.1f}, β1={beta_1_hat:.1f})", color=color_ols)
fig.show()
# # 3d plot --------------------
# from matplotlib import cm
# fig, ax = plt.subplots(dpi=120, subplot_kw={"projection": "3d"})
# surf = ax.plot_surface(Beta0, Beta1, Loss, rstride=1, cstride=1, linewidth=1, antialiased=True, cmap=cm.coolwarm)
# fig.colorbar(surf, shrink=0.5, aspect=5, pad=0.11)
# ax.set(xlabel="beta_0", ylabel="beta_1", zlabel="Residual Sum of Squares")
# # # add a point
# # ax.scatter(beta_0_hat, beta_1_hat, loss_, color="darkorange")
# # ax.text(beta_0_hat * 0.99, beta_1_hat, loss_ * 1.1, f"Lowest point (β0={beta_0_hat:.1f}, β1={beta_1_hat:.1f})", color="darkorange")
# fig.show()
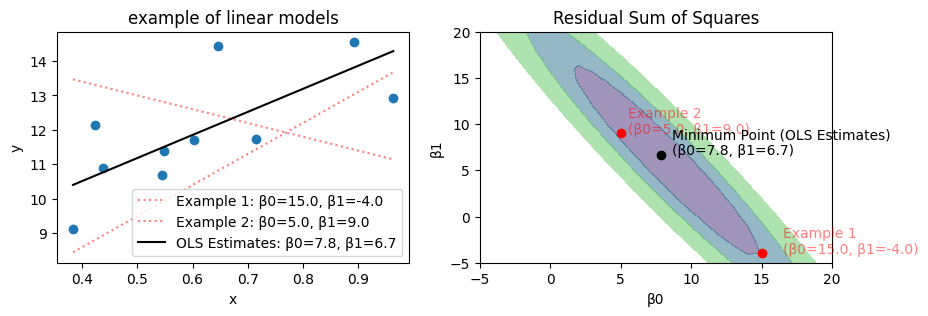
重回帰モデル#
説明変数を複数にしたものを重回帰モデルという。
モデル#
被説明変数を
を
と書くことができる
パラメータの推定#
残差二乗和を行列で表記すると
これを微分すると
となるため、これが0となるような
となる