フーリエ解析#
フーリエ解析(Fourier analysis):信号を異なる周波数の正弦波(sin)の重ね合わせとして表現すること
例えば画像を周波数の表現にして、周波数をフーリエ解析で分解したりする。
フーリエ級数#
幅
フーリエ係数は
である。 ここで
である。これを 基本周波数 といい、フーリエ級数は関数
Tip
基本周波数
例えば
複素数の指数関数#
複素数#
複素数とは
Show code cell source
import matplotlib.pyplot as plt
fig, ax = plt.subplots(figsize=(4, 3))
ax.arrow(-0.5, 0, 1.7, 0, head_width=0.05, head_length=0.1, fc='black', ec='black', length_includes_head=True)
ax.arrow(0, -0.5, 0, 1.5, head_width=0.05, head_length=0.1, fc='black', ec='black', length_includes_head=True)
ax.text(1.3, 0, "Re", fontsize=14, color='black')
ax.text(0, 1.1, "Im", fontsize=14, color='black')
ax.set_aspect('equal', adjustable='datalim')
ax.spines['top'].set_visible(False)
ax.spines['right'].set_visible(False)
ax.spines['left'].set_visible(False)
ax.spines['bottom'].set_visible(False)
ax.set(xticks=[], yticks=[])
c = 1 + 0.5j # 複素数
complex_points = [c, c.conjugate()] # conjugate: 共役複素数
colors = ['red', 'blue']
for i, (point, color) in enumerate(zip(complex_points, colors)):
x, y = point.real, point.imag
ax.plot([0, x], [0, y], color=color, linestyle='--', linewidth=0.8)
ax.scatter([x], [y], color=color)
z = r"$z$" if i == 0 else r"$\bar{z}$"
ax.text(x + 0.05, y + 0.05, f'{z} = ${point}$', fontsize=10, color=color)
plt.show()
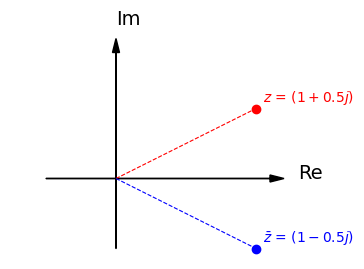
共役複素数#
複素数
となる。また重要な関係として
が成り立つ。
オイラーの式#
指数部が虚数の指数関数
これは オイラーの式 と呼ばれる式で、複素平面の単位円上の実軸から角度
が成り立つ。
証明
定義より次のように変形できる
両者が等しいことは、三角関数の加法定理
よりわかる
が成り立つ。
証明
オイラーの式 およびその式の
を使うと、
より
が得られる。また
より
が得られる。
フーリエ級数の複素表示#
を使うと、フーリエ級数は以下のように表すことができる。
ただし
である
また、
この
Show code cell source
import numpy as np
import matplotlib.pyplot as plt
fig, ax = plt.subplots(figsize=(4, 3))
# 軸を描画
ax.arrow(-1.2, 0, 2.4, 0, head_width=0.05, head_length=0.1, fc='black', ec='black', length_includes_head=True)
ax.arrow(0, -1.2, 0, 2.4, head_width=0.05, head_length=0.1, fc='black', ec='black', length_includes_head=True)
ax.text(1.3, 0, "Re", fontsize=14, color='black')
ax.text(0, 1.3, "Im", fontsize=14, color='black')
ax.set_aspect('equal', adjustable='datalim')
ax.spines['top'].set_visible(False)
ax.spines['right'].set_visible(False)
ax.spines['left'].set_visible(False)
ax.spines['bottom'].set_visible(False)
ax.set(xticks=[], yticks=[])
# 単位円
theta = np.linspace(0, 2*np.pi, 100)
x = np.cos(theta)
y = np.sin(theta)
plt.plot(x, y, 'k-', linewidth=1) # 黒い実線で単位円を描画
# curve
t = 0.3*np.pi
theta = np.linspace(0, t, 100)
x = np.cos(theta) * 0.2
y = np.sin(theta) * 0.2
ax.plot(x, y, 'k--', linewidth=1) # 黒い実線で単位円を描画
# text
m = len(x) // 2
ax.text(x[m], y[m], r"$\theta$")
# line
x = np.cos(t)
y = np.sin(t)
ax.plot([0, x], [0, y], color="blue", linestyle='-.', linewidth=0.8)
ax.scatter([x], [y], color="blue")
plt.show()
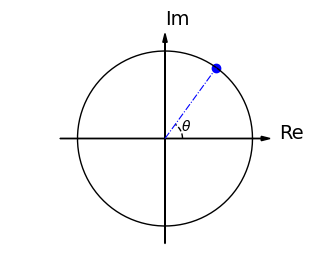
フーリエ変換#
フーリエ級数
において
とおき、
と書くと、
となる。周期
を信号
を 逆フーリエ変換 と呼ぶ。
Note
信号
(逆フーリエ変換)は周波数 の正弦波 を負の数を含めたすべての実数 に対して重ね合わせているフーリエ変換
は信号 に の共役複素数 を掛けて、すべての時刻 で積分した形になっている
フーリエ変換
逆フーリエ変換
逆フーリエ変換は信号
図:フーリエ変換のイメージ。
Show code cell source
import numpy as np
import matplotlib.pyplot as plt
# サンプルデータを生成する
N = 1000
d = 0.0001 # サンプリング周期
t = np.arange(0, N * d, d) # 時間
def f(t):
freq1, freq2 = 100, 500 # 周波数
s1, s2 = 1.5, 2 # スペクトル F(ω)
return s1 * np.sin(2 * np.pi * t * freq1) + s2 * np.sin(2 * np.pi * t * freq2)
y = f(t)
F = np.fft.fft(y) # フーリエ変換
freq = np.fft.fftfreq(N, d=d) # 周波数スケール
# 振幅スペクトル Amplitude の取得
# 振幅スペクトルは信号をフーリエ変換した結果の絶対値をとったもの
Amp = np.abs(F)
Amp = Amp / (N / 2) # 正規化
# 結果をプロット
fig, axes = plt.subplots(nrows=2)
axes[0].plot(t, y)
axes[0].set(title=r'Original Function $f(t)$', xlabel=r"$t$", ylabel=r"f(t)")
# 左右対称なので正の値だけ N//2 で取り出す
axes[1].stem(freq[:N//2], Amp[:N//2], 'r', markerfmt=" ", basefmt=" ")
axes[1].set(title=f'Absolute of Fourier Transform $|F(\omega)|$', xlabel=r'Frequency $ω$', ylabel=r'Magnitude $|F(ω)|$')
fig.tight_layout()
fig.show()
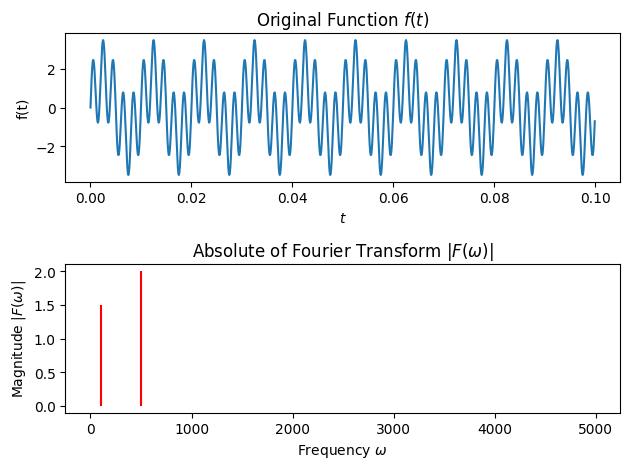
例:矩形窓#
次の関数は幅
このフーリエ変換は
となる。ただし、
と定義する。
Show code cell source
# 参考:numpyの高速フーリエ変換 fft を使う場合
# 自分で離散的なデータポイントからフーリエ変換するのは離散フーリエ変換を学んでからやる
import numpy as np
import matplotlib.pyplot as plt
fig, ax = plt.subplots(figsize=(4, 3))
# もとの関数 f(t)
w = 1.5 # width
def f(t):
return (1/2*w) * 1 * ((-w <= t) & (t <= w))
t = np.linspace(-3, 3, 100)
y = f(t)
ax.plot(t, f(t), label="f(t)", color="black", alpha=0.8)
# フーリエ変換 F(omega)
fourier = np.fft.fft(y)
inv_fourier = np.fft.ifft(fourier).real # 実部のみ
ax.plot(t, inv_fourier, label=r"$\hat{f}(f)$", color="red", linestyle="--", alpha=0.7)
ax.set(xlabel="t", ylabel="f(t)")
ax.legend()
plt.show()
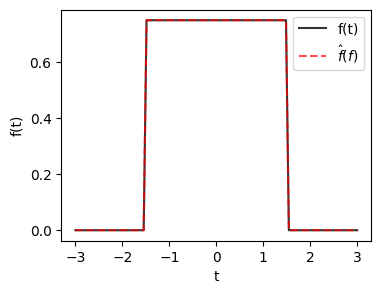
次の関数
このフーリエ変換は
ここで
となる
Show code cell source
from scipy import signal
import matplotlib.pyplot as plt
window = signal.windows.gaussian(51, std=7)
fig, ax = plt.subplots(figsize=(4, 2))
ax.plot(window)
ax.set(title=r"Gaussian window ($\sigma$=7)", ylabel="Amplitude", xlabel="Sample")
fig.show()
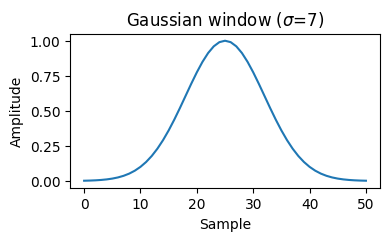
Show code cell source
import numpy as np
from scipy import signal
from scipy.fft import fft, fftshift
import matplotlib.pyplot as plt
fig, ax = plt.subplots(figsize=(4, 2.4))
window = signal.windows.gaussian(51, std=7)
A = fft(window, 2048) / (len(window)/2.0)
freq = np.linspace(-0.5, 0.5, len(A))
response = 20 * np.log10(np.abs(fftshift(A / abs(A).max())))
ax.plot(freq, response)
ax.plot(window)
ax.set(title=r"Frequency response of the Gaussian window ($\sigma$=7)",
ylabel="Normalized magnitude [dB]", xlabel="Normalized frequency [cycles per sample]",
xlim=(-0.5, 0.5), ylim=(-120, 0))
fig.show()
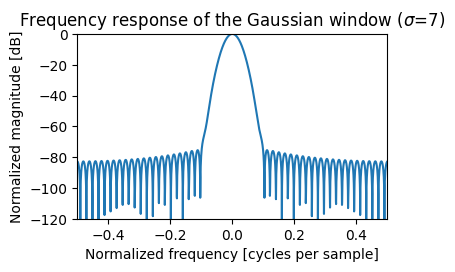
畳み込み積分#
たたみこみ積分
次の積分を信号
(左辺の積分における
畳み込み積分は分配法則、交換法則、結合法則が成り立つ
分配法則:
交換法則:
結合法則:
定理(信号の合成積はフーリエ変換の積)
信号
証明
(積分の順序を入れ換え、
一般に、ある集合に要素間の演算が定義されているものを 代数系 と呼ぶ。そして2つの異なる集合での演算が同じ規則に従うとき、2つの代数系は 同型 であるという。
関数の和
フィルター#
信号
矩形窓やガウス窓
これにより、関数
と書くことができる。
もし
その中間として、特定の
Show code cell source
import numpy as np
import matplotlib.pyplot as plt
N = 1000
t = np.linspace(0, 10, N)
def f(t):
return np.sin(t) + 0.5 * np.sin(10 * t)
signal = f(t)
fig, ax = plt.subplots(figsize=(4, 3))
ax.plot(t, signal, label="f(t)", color="black", alpha=0.8)
ax.set(xlabel="t", ylabel="f(t)")
from scipy.signal.windows import gaussian
from scipy.signal import fftconvolve
def gaussian_smoothing(signal, window_size, std_dev):
# ガウス窓を作成
gauss_window = gaussian(window_size, std=std_dev)
gauss_window /= gauss_window.sum() # 窓の正規化
# 畳み込み
return fftconvolve(signal, gauss_window, mode='same') # 同じ長さを保つ
smoothed_signal = gaussian_smoothing(signal, window_size=N//2, std_dev=100)
ax.plot(t, smoothed_signal, label=r"$\tilde{f}(t)$", color="steelblue", alpha=0.8)
# scipy
from scipy.ndimage import gaussian_filter1d
smoothed_signal = gaussian_filter1d(signal, 100)
ax.plot(t, smoothed_signal, label=r"$\tilde{f}(t)$ (scipy gaussian_filter1d)", color="orange", alpha=0.8)
ax.legend()
fig.show()
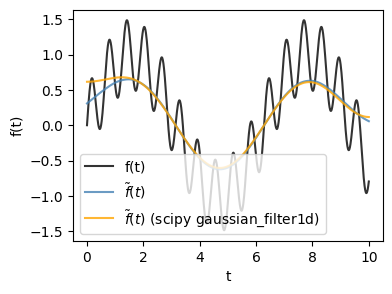
小まとめ
信号
して、個々の
そして窓関数
をすることで、特定の周波数をカットするなどの変換を行って信号を変換することができる。
一つの身近な具体例は画像に対するガウシアンフィルタ(ガウシアン平滑化・ガウシアンブラー)によるぼかし
パワースペクトル#
パーセバル(・プランシュレル)の式
信号
このとき、以下の パーセバル(・プランシュレル)の式 が成り立つ
証明
については、
が得られる
信号
パーセバルの第2式
はエネルギーがすべての
これを パワースペクトル と呼び、
は周波数
フーリエ変換
フーリエ変換は共役
Show code cell source
# うまくいかなかった
import numpy as np
import matplotlib.pyplot as plt
sampling_rate = 30
duration = 10
N = int(sampling_rate * duration)
t = np.linspace(0, duration, N, endpoint=False)
frequencies = [30, 60, 90, 150, 300]
amplitudes = [1.0, 0.8, 0.6, 0.4, 0.2]
signal = sum(
amp * np.sin(2 * np.pi * freq * t) for freq, amp in zip(frequencies, amplitudes)
)
# Perform the Fourier Transform
fft_result = np.fft.fft(signal)
frequencies = np.fft.fftfreq(len(signal), 1 / sampling_rate)
power_spectrum = np.abs(fft_result)**2
# 結果をプロット
fig, axes = plt.subplots(nrows=2)
axes[0].plot(t, signal)
axes[0].set(title=r'Signal $f(t)$', xlabel=r"$t$", ylabel=r"f(t)")
axes[1].stem(frequencies[:len(frequencies)], power_spectrum[:len(frequencies)])
# axes[1].stem(frequencies[:len(frequencies)//2], power_spectrum[:len(frequencies)//2])
axes[1].set(title=f'Power Spectrum $P(\omega)$', xlabel=r'Frequency $ω$', ylabel=r'Power Spectrum $P(ω)$')
fig.tight_layout()
fig.show()
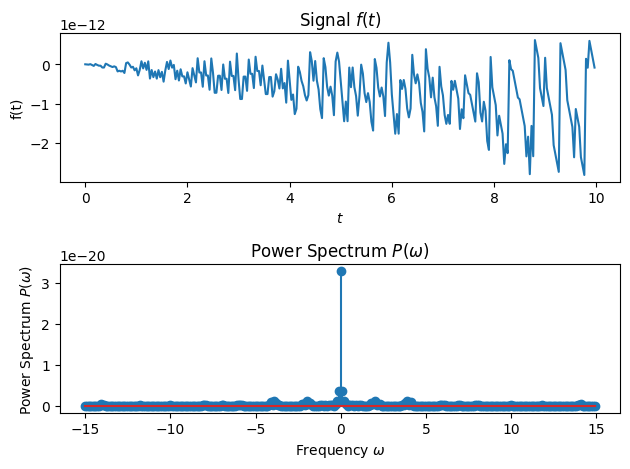
複素計量空間(ユニタリ空間)とのつながり#
信号
と定義すれば、ノルムも
と定義でき、フーリエ変換も
とおけば
となり、パーセバルの式は
とシンプルでエレガントな表記にすることができる。
この内積は計量ベクトル空間の内積の公理のうち対称性
(エルミート)内積
スカラとして複素数を取る線形空間
任意の
に対して . 等号が成り立つのは のときのみ ( 正値性 )任意の
に対して ( エルミート対称性 )任意の複素数
と任意の に対して ( 線形性 )
(ユニタリ)ノルム
ユニタリ空間(複素計量空間)
上記のような内積とノルムが定義されている複素線形空間
自己相関関数#
自己相関関数
次の関数
Show code cell source
import numpy as np
from scipy import signal
rng = np.random.default_rng()
sig = rng.standard_normal(100)
autocorr = signal.fftconvolve(sig, sig[::-1], mode='full')
import matplotlib.pyplot as plt
fig, (ax_orig, ax_mag) = plt.subplots(2, 1, dpi=72, figsize=[6, 4])
ax_orig.plot(sig)
ax_orig.set(title=f'White noise $f(t)$', xlabel=r"$t$")
ax_mag.plot(np.arange(-len(sig)+1,len(sig)), autocorr)
ax_mag.set(title=r'Autocorrelation $R(\tau)$', xlabel=r"$\tau$")
fig.tight_layout()
fig.show()

実数の信号
複素数の信号の場合はエルミート内積
このように、内積・エルミート内積は類似度の尺度となり、 (相互)相関 と呼ばれる。
証明
第1式は
で示される。また共役複素数をとっても絶対値は変化しないため第2式が成り立つ。
ウィーナー・ヒンチンの定理#
ウィーナー・ヒンチンの定理
自己相関関数のフーリエ変換はパワースペクトルに等しい。
よってパワースペクトルを算出するには、2通りの方法がある
信号
のフーリエ変換 を計算して絶対値の2乗を取る自己相関関数
を計算してフーリエ変換する
信号を精度よく積分するのは難しいため、自己相関関数で算出するほうが実用上は便利らしい
Show code cell source
import numpy as np
from scipy import signal
rng = np.random.default_rng(seed=0)
sig = rng.standard_normal(500)
autocorr = signal.fftconvolve(sig, sig[::-1], mode='full')
import matplotlib.pyplot as plt
fig, axes = plt.subplots(3, 1, dpi=80, figsize=[6, 5])
axes[0].plot(sig)
axes[0].set(title=f'White noise $f(t)$', xlabel=r"$t$")
n = len(sig)
x = np.arange(-n+1, n)
axes[1].plot(x, autocorr)
axes[1].set(title=r'Autocorrelation $R(\tau)$', xlabel=r"$\tau$")
# Power spectrum
omega, P = signal.periodogram(sig, window='flattop', scaling='spectrum')
axes[2].semilogy(omega, P)
axes[2].set(title=r'Power spectrum $P(\omega)$', xlabel=r"$\omega$")
fig.tight_layout()
fig.show()
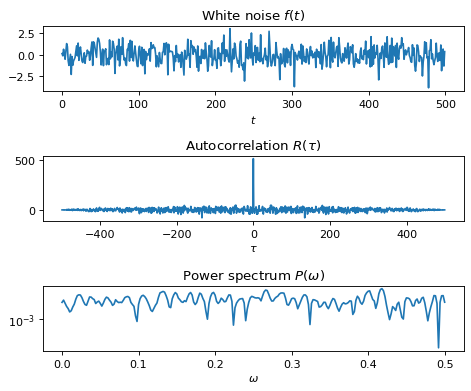
Show code cell source
from scipy import signal, fft
import matplotlib.pyplot as plt
fig, axes = plt.subplots(2, 1, dpi=80, figsize=[6, 4])
axes[0].plot(sig)
axes[0].set(title=f'White noise $f(t)$', xlabel=r"$t$")
n = len(sig)
T = 1
F = fft.fft(sig, len(sig))[:n//2]
omega = fft.fftfreq(n, T)[:n//2]
P = np.abs(F) ** 2
# Power spectrum from F(ω)
axes[1].semilogy(omega, P)
axes[1].set(title=r'Power spectrum $P(\omega)$ from $F(\omega)$', xlabel=r"$\omega$")
fig.tight_layout()
fig.show()
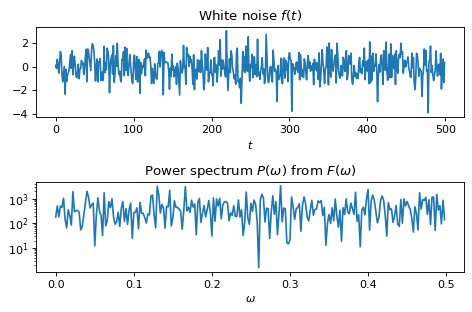
それっぽいやつやってみたがうまくPが一致しない
そもそもホワイトノイズのPは1のはず…
サンプリング定理#
信号
次の性質を持つ関数
補間関数の例は
Show code cell source
import numpy as np
import matplotlib.pyplot as plt
def sinc(x):
return np.sin(np.pi * x) / (np.pi * x)
fig, ax = plt.subplots(figsize=[4,2])
x = np.linspace(-3*np.pi, 3*np.pi, 100)
ax.plot(x, sinc(x))
ax.set(title="sinc(x)", xlabel="x", ylabel="sinc(x)")
fig.show()
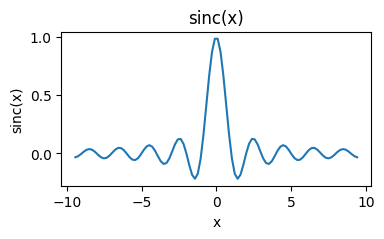
はすべてのサンプル点
定義:帯域制限
信号
であれば、信号
サンプリング間隔
Show code cell source
import numpy as np
import matplotlib.pyplot as plt
fig, ax = plt.subplots(figsize=[4,2])
x = np.linspace(-2*np.pi, 2*np.pi, 100)
ax.plot(x, np.sin(x))
ax.set(title="sin(x)", xlabel="x", ylabel="sin(x)")
ax.axhline(0, color="black", alpha=0.7)
ax.axvline(0, color="darkorange", linestyle="--", alpha=0.7)
ax.axvline(np.pi, color="darkorange", linestyle="--", alpha=0.7)
ax.text(np.pi / 2, -0.5, r"$\tau$", color="darkorange", ha="center")
fig.show()
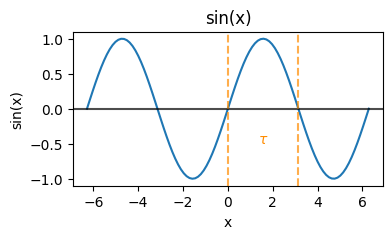
サンプリング定理
帯域幅
のサンプル点
証明
信号
となる。
区間
よって
となる。
まとめると、
一定の条件を満たす間隔で得られたサンプルと、一定の条件を満たす信号は、 離散的なサンプルからもとの連続的な信号を再現できる というのがサンプリング定理。
音声などの信号に限らず、デジタル画像などアナログ(連続値)なものをデジタル(離散値)にサンプリングする分野で広く応用されている。
間隔
Show code cell source
import numpy as np
import matplotlib.pyplot as plt
def approx(x, tau):
# tau: サンプリング周期
# 間隔を指定してのサンプリングが難しかったのでarangeで生成
x_samples = np.arange(-3 * np.pi, 3 * np.pi, tau)
y_samples = np.sin(x_samples)
y_hat = []
for i in range(len(x)):
y_hat_i = 0
for j in range(len(y_samples)):
y_hat_i += y_samples[j] * np.sinc((x[i] - x_samples[j]) / tau)
y_hat.append(y_hat_i)
return x_samples, y_samples, np.array(y_hat)
taus = [
("\pi", np.pi),
("\pi/1.1", np.pi/1.1),
("\pi/2", np.pi/2),
]
fig, axes = plt.subplots(figsize=[12, 2.5], ncols=len(taus), sharey=True)
for i, ax in enumerate(axes):
# true signal
x = np.arange(-3 * np.pi, 3 * np.pi, 0.1)
y = np.sin(x)
ax.plot(x, y, label=r"true signal $f(t)$", color="black", alpha=0.7)
# approximate
tau_str, tau = taus[i]
x_samples, y_samples, y_hat = approx(x, tau=tau)
ax.plot(x_samples, y_samples, label=r"samples", alpha=0.8, linestyle=":", marker=".")
ax.plot(x, y_hat, label=rf"approximation ($\tau = {tau_str}$)", alpha=0.8, linestyle="--")
ax.set(xlabel=r"$t$", title=rf"Approximation ($\tau = {tau_str} = {tau:.2f}$)")#, ylim=(-1.2, 3.2))
ax.legend()
if i == 0:
ax.set(ylabel=r"$f(t)$")
fig.show()
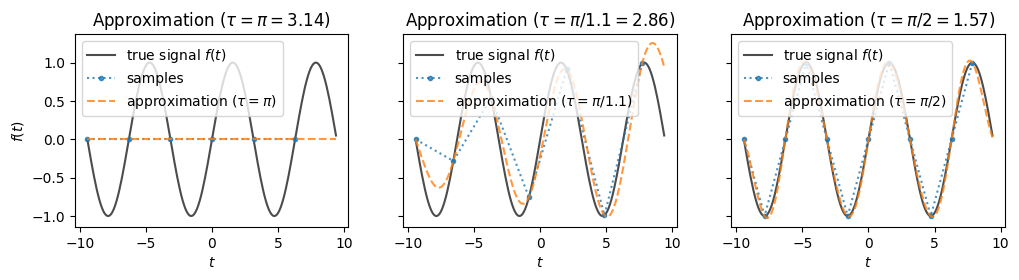
周期
1周期が
参考#
金谷健一. (2003). これなら分かる応用数学教室: 最小二乗法からウェーブレットまで.