一致性のシミュレーション#
標本平均の漸近分布#
概要#
標本平均
これは中心極限定理により
となる。式を整理して表現を少し変えると
である。
と表すこともできる。このことを「
シミュレーション#
モンテカルロシミュレーションで標準誤差を確かめてみる
標準誤差が推定量
Show code cell source
import numpy as np
import matplotlib.pyplot as plt
# generate data
np.random.seed(0)
mu = 10
sigma = 2
n_iter = 10000
results = []
for n in [10, 100, 1000]:
means = []
errors = []
for i_iter in range(n_iter):
samples = np.random.normal(loc=mu, scale=sigma, size=n)
# 標本平均 \bar{X}
means.append(samples.mean())
# 標準化した推定誤差
mu_hat = samples.mean()
error = np.sqrt(n) * (mu_hat - mu)
errors.append(error)
results.append({
"n": n,
"means": means,
"errors": errors
})
# plot
fig, ax = plt.subplots()
for result in results:
n = result["n"]
means = result["means"]
ax.hist(means, label=f"{n=} mean={np.mean(means):.1f} s.e.={np.std(means):.2f} | " + r"estimate $\sigma/\sqrt{n}$" + f"={sigma/np.sqrt(n):.2f}", alpha=0.7, bins=30)
ax.legend()
ax.set(xlabel=r"$\bar{X}$", title=fr"distribution of sample mean $\bar X$ ($X \sim N$({mu}, {sigma**2}))")
fig.show()
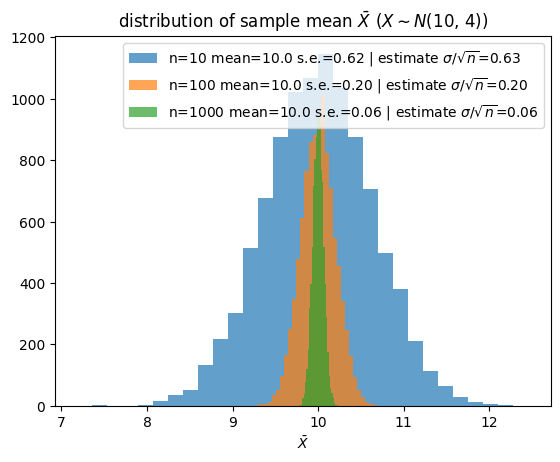
標準化した推定誤差
こちらは
となっていることがわかる。
Show code cell source
# plot
fig, ax = plt.subplots()
for result in results:
n = result["n"]
errors = result["errors"]
ax.hist(errors, label=f"{n=} mean={np.mean(errors):.3f} var={np.var(errors):.2f}", alpha=0.5, bins=30)
ax.legend()
ax.set(xlabel=r"$\sqrt{n}(\bar{X} - \mu)$",
title=r"$\sqrt{n}(\bar{X} - \mu) \to N(0, \sigma^2)$")
fig.show()
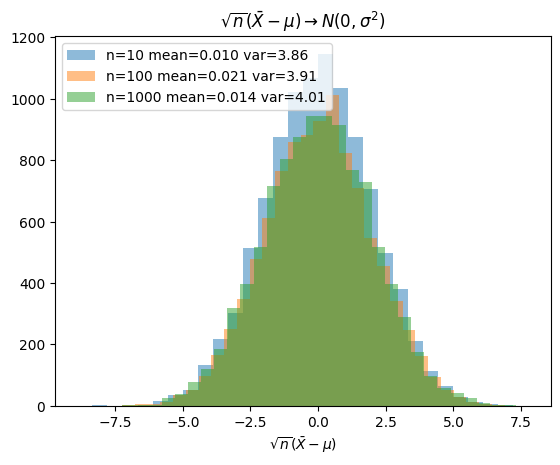
二項分布の場合#
Show code cell source
import numpy as np
import matplotlib.pyplot as plt
# generate data
np.random.seed(0)
p = 0.5
mu = p
sigma = p * (1-p)
n_iter = 10000
results = []
for n in [10, 30, 100, 384, 1000]:
means = []
errors = []
for i_iter in range(n_iter):
samples = np.random.binomial(n=1, p=mu, size=n)
# 標本平均 \bar{X}
means.append(samples.mean())
# 標準化した推定誤差
mu_hat = samples.mean()
error = np.sqrt(n) * (mu_hat - mu)
errors.append(error)
results.append({
"n": n,
"means": means,
"errors": errors
})
# plot
fig, ax = plt.subplots()
for result in results:
n = result["n"]
means = result["means"]
ax.hist(means, label=f"{n=} mean={np.mean(means):.1f} s.e.={np.std(means):.2f} | " + r"estimate $\sigma/\sqrt{n}$" + f"={sigma/np.sqrt(n):.2f}", alpha=0.7, bins=30)
ax.legend()
ax.set(xlabel=r"$\bar{X}$", title=fr"distribution of sample mean $\bar X$ ($X \sim Binom$(n=1, p={p}))")
fig.show()
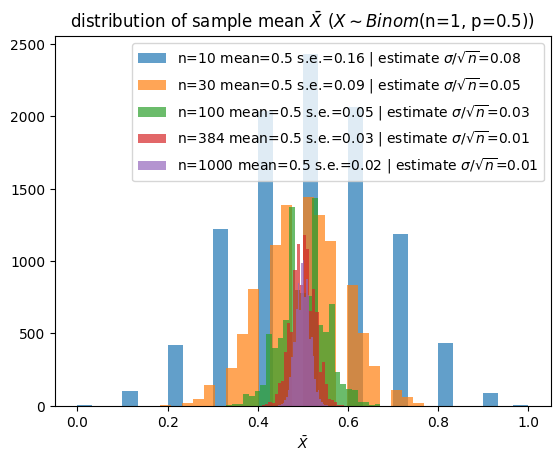