線形判別モデル#
モデル#
2クラスの場合#
2クラス\((C_1, C_2)\)を識別する線形モデルを考える。
特徴量ベクトルを\(\boldsymbol{x}=(x_1, \cdots, x_d)^\top\)、係数ベクトルを\(\boldsymbol{w}=(w_1, \cdots, w_d)^\top\)、バイアス項を\(w_0\)とすれば、
で表される。
識別境界を\(f(\boldsymbol{x})=0\)として、\(f(\boldsymbol{x})=0\)のときはリジェクトせずに\(C_1\)とする場合、予測値\(\hat{C}\)を出力する識別規則は
となる。
多クラスの場合#
クラス数が\(K(>2)\)個ある場合にはどうすればよいだろうか。
いくつか方法はある(はじパタ 6.1.2などを参照)が、最大識別関数法が現状もっとも良さそう。
これは\(K\)個の線形識別関数\(f_j(\boldsymbol{x}) \ (j = 1, 2, \cdots, K)\)を用意して、最も出力値が大きいクラスを採用するというもの。
パラメータの推定#
最小二乗誤差基準#
係数ベクトルにバイアスを含めて\(\boldsymbol{w}=(w_0, w_1, \cdots, w_d)^\top\)とし、特徴量ベクトルを\(\boldsymbol{x}=(1, x_1, \cdots, x_d)^\top\)と表記することにする。
それにより、線形識別関数を
と表記する。
教師ラベルは\(\{+1, -1\}\)で表現されるものとする。
ここで\(i\)はサンプルの添字で\(i = 1, \cdots, N\)である。
特徴量を行列\(\boldsymbol{X} = (\boldsymbol{x}_1, \cdots, \boldsymbol{x}_N)^\top\)、教師ラベルのベクトルを\(\boldsymbol{t}=(t_1, \cdots, t_N)^\top\)と表記する。
二乗誤差\(E(\boldsymbol{w})\)を使って評価すると、次のようになる。
二乗誤差を最小にするパラメータ\(\boldsymbol{w}\)はパラメータで微分して0になるパラメータなので、
を解くことにより
である。
実装#
以下のようなデータがあったとする
Show code cell source
# 2次元に描いた場合
import numpy as np
import matplotlib.pyplot as plt
# このようなデータがあったとする
x_c1 = np.array([
[.50, .55],
[.45, .75],
[.7, .50],
[.7, .75],
])
x_c2 = - x_c1
X = np.append(x_c1, x_c2, axis=0)
y = np.array([1] * x_c1.shape[0] + [-1] * x_c2.shape[0])
def plot_hyperplane(X, y, ax):
# データ点の散布図
is_1 = y == 1
ax.scatter(X[is_1, 0], X[is_1, 1], label="C1", color="orange")
ax.scatter(X[~is_1, 0], X[~is_1, 1], label="C2", color="steelblue")
# # x軸, y軸を描く
# ax.hlines(0, -1, 1, colors="black", linewidth=1)
# ax.vlines(0, -1.1, 1.1, colors="black", linewidth=1)
ax.legend(loc="upper left")
return ax
fig, ax = plt.subplots()
plot_hyperplane(X, y, ax)
ax.set_xlim(-1, 1)
ax.set_ylim(-1, 1)
fig.show()
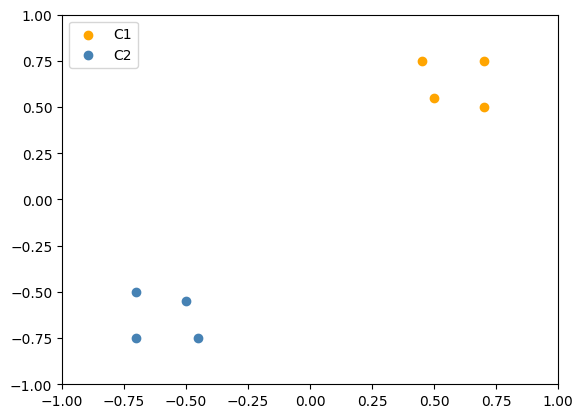
# 最小二乗法によるパラメータの推定
w = np.linalg.inv(X.T @ X) @ X.T @ y
w
array([0.78271718, 0.82605555])
このパラメータによる識別超平面を描くと次の図のようになる
Show code cell source
# 描画範囲
x1 = np.linspace(-1, 1, 100)
# 超平面:x2 = -(w1/w2) * x1の形にする
x2 = -(w[0] / w[1]) * x1
# plot
fig, ax = plt.subplots()
plot_hyperplane(X, y, ax)
ax.plot(x1, x2, color="dimgray", label="f(x)=w'x")
ax.legend(loc="upper left")
fig.show()
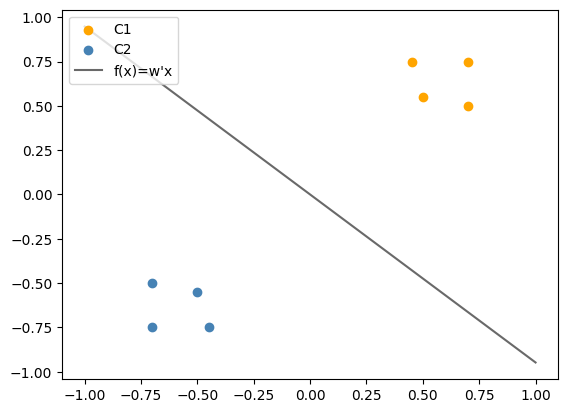