OLSの検定・区間推定#
OLS推定量の標準誤差#
ある推定量の漸近分布(asymptotic distribution、大標本において推定量が近似的に従う分布)の標準偏差を標準誤差(standard error)という。
重回帰モデル
において、各
が近似的に標準正規分布
ここで
上記の式は書き換えると
となる。ここで
とおけば、
別の定義では、残差を
で、
誤差項
分散が既知かつ不均一分散のとき
(『統計的因果推論の理論と実装』p.118)
b = 1
np.exp(b) - 1
1.718281828459045
model.summary()
Dep. Variable: | y | R-squared: | 0.675 |
---|---|---|---|
Model: | OLS | Adj. R-squared: | 0.674 |
Method: | Least Squares | F-statistic: | 619.8 |
Date: | Fri, 04 Apr 2025 | Prob (F-statistic): | 9.04e-75 |
Time: | 10:54:42 | Log-Likelihood: | -422.93 |
No. Observations: | 300 | AIC: | 849.9 |
Df Residuals: | 298 | BIC: | 857.3 |
Df Model: | 1 | ||
Covariance Type: | nonrobust |
coef | std err | t | P>|t| | [0.025 | 0.975] | |
---|---|---|---|---|---|---|
Intercept | 10.0882 | 0.117 | 86.551 | 0.000 | 9.859 | 10.318 |
x | 4.9289 | 0.198 | 24.896 | 0.000 | 4.539 | 5.318 |
Omnibus: | 1.176 | Durbin-Watson: | 2.115 |
---|---|---|---|
Prob(Omnibus): | 0.555 | Jarque-Bera (JB): | 1.153 |
Skew: | -0.033 | Prob(JB): | 0.562 |
Kurtosis: | 2.703 | Cond. No. | 4.42 |
Notes:
[1] Standard Errors assume that the covariance matrix of the errors is correctly specified.
# CI
ci = (
beta1 - 1.96 * se_beta1,
beta1 + 1.96 * se_beta1
)
fig, ax = plt.subplots()
x_plot = np.linspace(4, 6, 50)
y_plot = norm.pdf(x=x_plot, loc=beta1, scale=se_beta1)
ax.plot(x_plot, y_plot, alpha=.5)
ax.axvline(ci[0])
ax.axvline(ci[1])
<matplotlib.lines.Line2D at 0x7f1a7b0202e0>
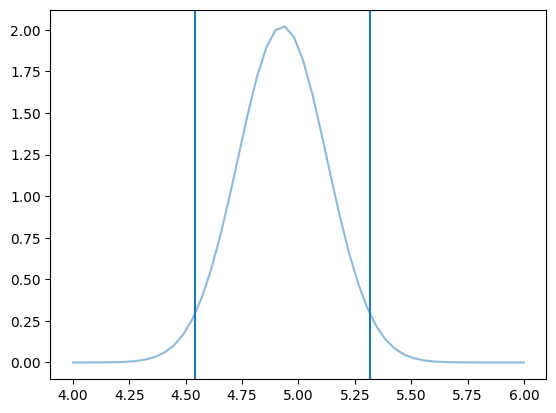
Note
データによる例
例えば次のように生成されたデータがあるとする。
シミュレーションによって(データを生成して推定することを繰り返すことによって)この分布を見てみる
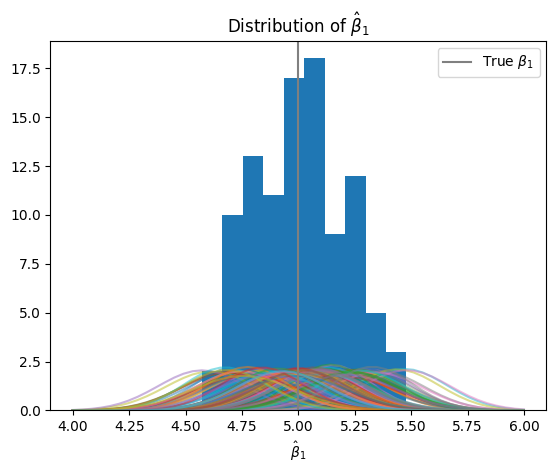
OLS推定量の 検定#
帰無仮説と対立仮説をそれぞれ
とする。
と定義すると、これは標準正規分布
検定の 値#
帰無仮説
OLS推定量の信頼区間#
は
と近似できる。そこから95%信頼区間は
となる
回帰の標準誤差#
回帰の標準誤差(standard error of the regression: SER)は、回帰誤差
(
ここで